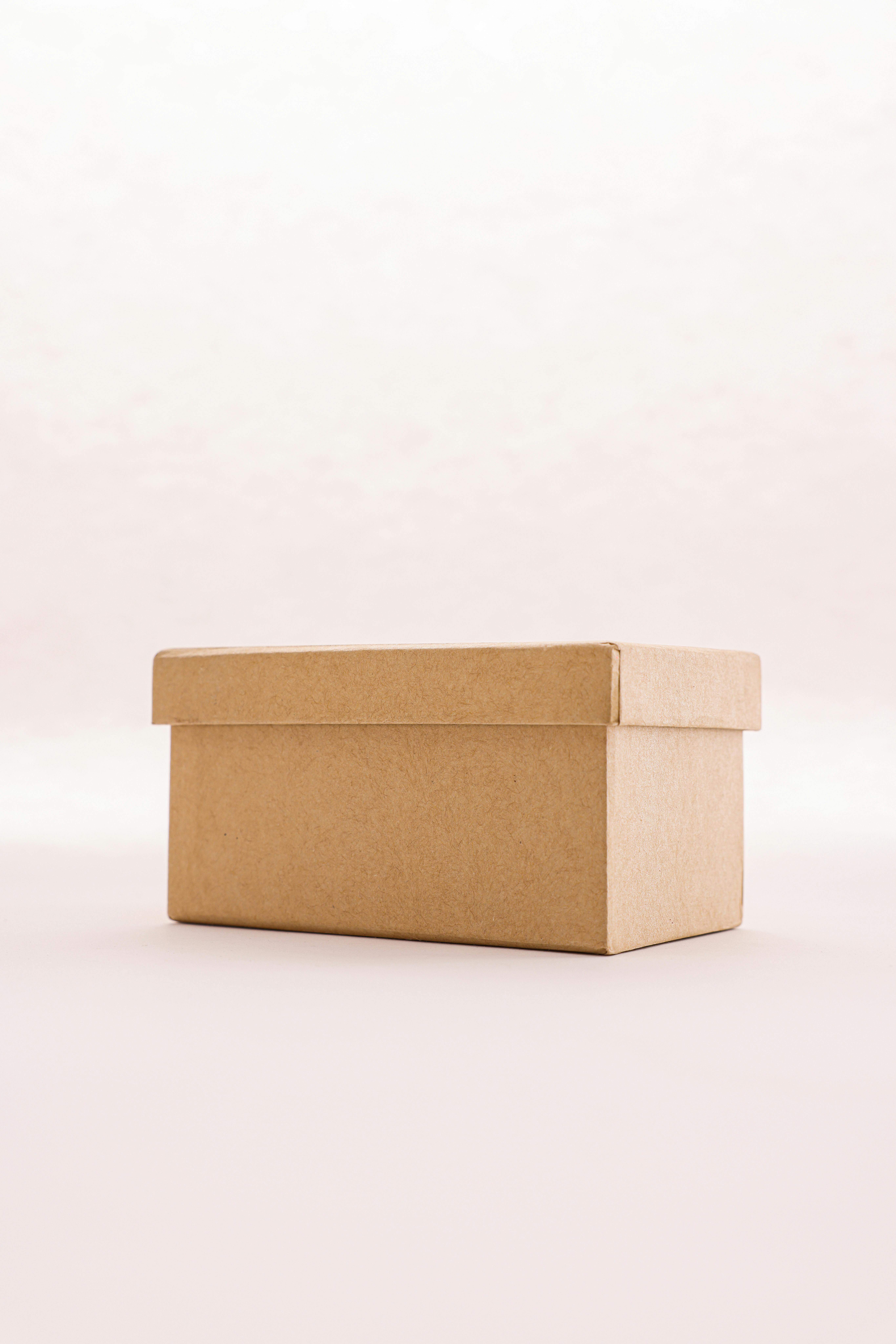

AI-powered order management system and supply chain synchronization
The rapid advancement of artificial intelligence (AI) is transforming supply chain management, particularly in the synchronization of order management systems (OMS) with logistics networks. AI-powered solutions enable real-time communication, predictive analytics, and automated decision-making to enhance efficiency, reduce errors, and optimize workflows. These innovations are essential for any b2b ecommerce platform looking to streamline b2b inventory management and improve supply chain planning.
How AI improves OMS and supply chain communication
- Real-time data processing: AI facilitates seamless data exchange between OMS and supply chain networks, ensuring that inventory levels, demand forecasts, and order statuses are updated in real time (Ivanov & Dolgui, 2021). This is particularly relevant for enterprise ecommerce platforms that require accurate stock updates.
- Predictive analytics: Machine learning algorithms analyze historical and real-time data to forecast demand fluctuations, allowing businesses to adjust procurement and production accordingly (Choi et al., 2022). This contributes to ecommerce automation, reducing human intervention and errors.
- Automated order fulfillment: AI enhances automation in warehousing and logistics, reducing manual intervention and improving order accuracy (Speranza, 2018). This is a key part of any b2b ecommerce strategy.
- AI-driven decision support: AI assists supply chain managers in making data-driven decisions, such as rerouting shipments to avoid bottlenecks or identifying the best fulfillment center for an order (Min, 2022). This level of efficiency is crucial for b2b wholesale operations.
Case study: Amazon’s AI-integrated supply chain
Amazon’s AI-driven fulfillment system integrates machine learning to predict demand, manage inventory, and optimize last-mile delivery. This results in faster shipping, reduced costs, and increased customer satisfaction (Brynjolfsson & McAfee, 2017), setting a benchmark for b2b marketplace optimization.
Reducing delivery delays with AI in order management
Delivery delays pose a significant challenge for businesses and consumers. AI enhances order management by predicting potential disruptions and proactively rerouting shipments to ensure timely deliveries, a must-have feature for any ecommerce growth strategy.
AI’s role in reducing delays
- Demand-supply forecasting: AI predicts demand spikes and adjusts inventory allocation to prevent stockouts and overstock situations (Fahimnia et al., 2015). This supports supply chain planning for b2b sales.
- Dynamic routing optimization: AI-powered route optimization systems adjust delivery routes in real-time based on traffic patterns, weather conditions, and unforeseen disruptions (Tan et al., 2020). B2b shipping providers use this to ensure efficient logistics.
- Proactive issue resolution: AI-driven anomaly detection identifies potential delays by analyzing logistics data and triggers automated contingency plans (Baryannis et al., 2019). This is critical for b2b partner programs that rely on seamless logistics.
- Autonomous last-mile delivery: AI integrates with autonomous vehicles and drones to improve last-mile delivery efficiency, reducing reliance on human drivers (Winkenbach, 2021). This technology plays a role in b2b digital transformation.
Example: DHL’s AI-enhanced logistics
DHL uses AI-powered predictive analytics to anticipate shipment delays, allowing the company to take preemptive action. This has improved on-time deliveries and enhanced customer satisfaction (Christopher, 2016). Such advancements can also aid in b2b payment processing by ensuring smoother transactions and accurate delivery estimates.
AI-driven sustainability in order management
Sustainability is a growing concern in supply chain management. AI-driven solutions optimize packaging, routing, and fulfillment strategies to minimize environmental impact while aligning with b2b ecommerce trends.
AI’s contribution to sustainable order management
- Eco-friendly packaging optimization: AI analyzes order compositions to recommend minimal and sustainable packaging materials, reducing waste (Björklund et al., 2016). This is crucial for b2b product marketing and responsible supply chain operations.
- Carbon footprint reduction via route optimization: AI-powered logistics software minimizes fuel consumption by selecting the most efficient delivery routes (McKinnon et al., 2018), an essential feature for b2b lead generation strategies focused on sustainability.
- Smart warehousing for energy efficiency: AI optimizes warehouse layouts and automation to reduce energy consumption (Van der Vorst & Beulens, 2018). This contributes to ecommerce security and compliance.
- AI-enabled reverse logistics: AI streamlines return processes, promoting circular economy practices and reducing landfill waste (Govindan et al., 2015), a feature that enhances b2b customer experience.
Example: UPS’s AI-driven sustainability initiatives
UPS employs AI in its ORION (On-Road Integrated Optimization and Navigation) system to optimize delivery routes, reducing fuel consumption and CO2 emissions significantly (Speranza, 2018). This innovation can serve as a model for b2b personalization in logistics.
AI’s integration into supply chain and logistics management is revolutionizing order management, reducing delays, and promoting sustainability. Companies leveraging AI-powered OMS and logistics synchronization gain competitive advantages by improving efficiency, reducing costs, and meeting environmental goals. This is critical for companies engaged in digital transformation and those leveraging ecommerce personalization to optimize operations.
References
- Baryannis, G., Dani, S., & Antoniou, G. (2019). Predictive analytics for supply chain risk management. International Journal of Production Research, 57(7), 2212-2229.
- Björklund, M., Martinsen, U., & Abrahamsson, M. (2016). Sustainability in logistics and supply chain management. Business Strategy and the Environment, 25(6), 389-401.
- Brynjolfsson, E., & McAfee, A. (2017). Machine, Platform, Crowd: Harnessing Our Digital Future. W. W. Norton & Company.
- Choi, T. M., Wallace, S. W., & Wang, Y. (2022). Big data analytics in operations management. Production and Operations Management, 31(3), 1201-1215.
- Christopher, M. (2016). Logistics & Supply Chain Management. Pearson.